Serving Up Value By Controlling Food and Beverage Processes
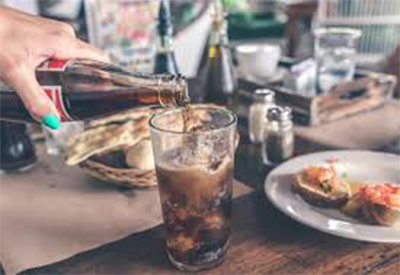
June 11, 2019
By Vincent Heng, Senior Application Engineer, Rockwell Automation
As food processing continues to grow in complexity, companies remain vigilant for how to make facilities work better without expanding their footprint. When considering investments that can make the next leap in productivity, the solution is often cultural or technological.
Employees, for instance, might receive additional training to learn the best way to work a recipe, so wouldn’t consider how technology could move them forward. Or collecting more data from the fryer might promise insight into performance but doesn’t add the context about what happens next in the process.
The question of how people and technology work together is especially complex when going through a digital transformation. Finding the place to start for making improvements requires identifying those capabilities that solve operational needs.
Model predictive control (MPC) is one such technology. It manages minute details and adjustments across processes, helping operators optimize production while maintaining or improving quality.
A common thread through the culture of many food processors is a trust that operators know what they’re doing. They’ve worked an area or particular process for years, so they know how to manipulate the process. They have earned the freedom to tinker as they see fit. And for their role, they do great work.
The challenge is the natural limits you hit when making manual adjustments. After all, it’s difficult to have the right operator in the right place at the right time to catch issues, make the necessary manual adjustments and stay in place long enough to ensure success. Operators may also be running the same machines in different ways on different shifts.
A related challenge is that despite their experience, operators often specialize at their process area, which results in different groups that have a range of different organizational responsibilities. An operator working on the frying process may have different metrics for success than an operator working on freezing – and these metrics could be at odds with one another.
Ultimately, however, if everyone on the line is not working together, they can’t optimize overall capacity. The problem is only heightened by scale; most processors have more than one line, and each one may run differently even though they are functionally very similar.
MPC brings everyone together using a wealth of historic plant data to identify how each part should ideally be working. We call it a standard model for production, which monitors a variety of process metrics and continuously looks for opportunities to improve throughput up and down the line. It then provides the intelligence operators need to do their jobs in an enhanced capacity, making highly targeted adjustments that yield significant material benefits and hit more advanced quality metrics.
These models add particular value at key points in production where bottlenecks occur. In frozen french fry production, for instance, some of the most frequent bottlenecks include direct sorting, defect sorting and freezing.
Consider defect sorting: most raw potatoes have bruises substantial enough to be a defect. Once the potatoes are cut, the specifications only allow for a limited number of fries with these defects to go through. Machines sort by analyzing 900 or more fries per minute, taking those with defects out of the line – but they still may not catch enough.
To stay on specification and prevent the bags from containing too many defects, the line needs to be slowed down. MPC decreases the line rate proactively, if too many defects are making it through, boosting efficiency and protecting product quality.
Technologies like MPC help food and beverage manufacturers leverage the strengths and experience of their people while making the best use of advanced data analytics. Together, they support greater efficiency and improved capacity throughout the organization.
Learn more about MPC from Rockwell Automation.